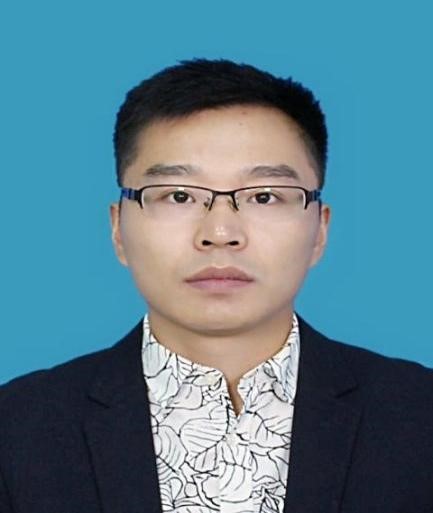
报告时间:2022年9月23日、9月30日、10月14 日、10月21日、10月28日(每周五上午10:00-11:00)
报告地点:腾讯会议ID:342-381-779(一); 189-495-797(二);343-534-277(三);
190-922-037(四);923-999-393(五);
报告人: Hongtian Chen 教授
工作单位:University of Alberta, Canada.
举办单位:欧洲杯买球官方官网
报告人简介:Hongtian Chen received his B.S. and M.S. degrees from the School of Electrical and Automation Engineering from the Nanjing Normal University, China, in 2012 and 2015, respectively. He received his doctorate from the College of Automation Engineering from the Nanjing University of Aeronautics and Astronautics, China, in 2019. He was a Visiting Scholar at the Institute for Automatic Control and Complex Systems, University of Duisburg-Essen, Germany, in 2018. Currently, he is a postdoctoral fellow with the Department of Chemical and Materials Engineering, University of Alberta, Canada. His research interests include process monitoring and fault diagnosis, data mining and analytics, machine learning, and quantum computation, and their application to high-speed trains, new energy systems, and industrial processes.
Dr. Chen was a recipient of the Grand Prize of the Innovation Award of the Ministry of Industry and Information Technology of the People’s Republic of China in 2019, the Excellent Ph.D. Thesis Award of Jiangsu Province in 2020, and the Excellent Doctoral Dissertation Award from the Chinese Association of Automation (CAA) in 2020. He serves as Associate Editor and Guest Editor for several international journals such as IEEE Transactions on Instrumentation and Measurement, IEEE Transactions on Artificial Intelligence, IEEE Transactions on Neural Networks and Learning Systems, Control Engineering Practice, and Reliability Engineering & System Safety. He was also an organizing chair and program chair for international conferences such as the 5th International Conference on Robotics, Control and Automation Engineering.
报告简介:In the report series, the fault diagnosis (FD) and fault-tolerant control (FTC) issues will be detailed from static to dynamic methods and from model-based to data-driven approaches. In the Part 1, the introduction of static FD approaches includes machine learning-based modeling and several test statistics. In order to have a insightful understanding of data-driven FD and FTC approaches (Part 4), model-based FD and FTC using an observer will come first respectively in Parts 2 and 3, which play an essential role in the data-driven versions. As both parameter and system identification are achieved using system data whose size must be limited, the few-shot learning-based strategy will be elaborated in Part 5, and its performance will also be considered. The subtitles of this report are given as follows.
1.Static Fault Diagnosis Approaches: Data-driven Designs
2.An Observer-based Fault Diagnosis Framework: Model-based Designs
3.An Observer-based Fault-tolerant Control Framework: Model-based Designs
4.Fault Diagnosis and Fault-tolerant Control for Dynamic Systems: Data-driven Designs
5.System Identification Using Few-shot Learning and Its Performance Evaluation
文 许水清 审核 赵吉文